AI Enters the Medical Field
It is well-known how useful AI is, but to what extent has it been used? Is it effective in sensitive fields like medicine? This article will discuss that in more detail and mention some examples of its impact.
ARTICLES
Abdullah Amro | Edited by I.T. Aras
3/20/20243 min read
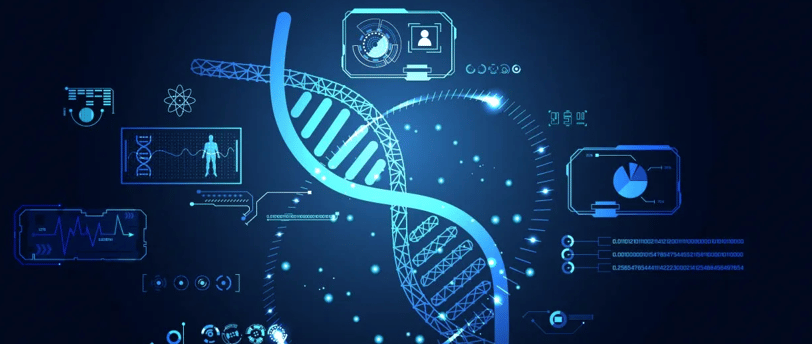
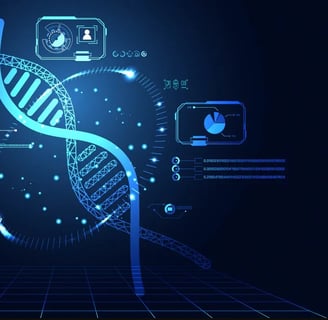
Artificial intelligence (AI) is playing an increasingly important role in healthcare. AI algorithms can now analyze large amounts of medical data to identify patterns and trends that would be difficult or impossible for humans to see. This information can then improve disease diagnosis, treatment, and prevention. Artificial Intelligence (AI) has emerged in healthcare in recent years, giving rise to innovations that promise to improve patient health outcomes and workflow efficiencies. From diagnosis to treatment and home care, AI has shown the potential to support healthcare professionals and patients at every stage of the care continuum. Here are five real-world examples of AI in healthcare, showing how it can enhance experiences for patients and staff alike.
1. Improving precision in patient positioning and CT image reconstruction
Radiology departments continue to face challenges in increasing patient volumes, ensuring consistency in image quality, and improving operational efficiency. With Computed Tomography (CT) being one of the most widely used imaging modalities, radiology departments have much to gain from AI-enabled solutions that help streamline CT workflows and maximize image quality.
That starts with getting a patient in the proper position for an exam. In CT, patient mispositioning is a common challenge, which can result in increased radiation dose to the patient or image noise. AI-enabled camera technology can automatically detect anatomical landmarks in a patient to enable fast, accurate, and consistent patient positioning. In addition, AI-enabled image reconstruction can help reduce radiation dose and improve CT image quality, thereby supporting diagnostic confidence.
2. Speeding up image acquisition in MR
Magnetic resonance (MR) is an increasingly important imaging modality in precision diagnosis of medical conditions. The rising use of MR increases the pressure to efficiently scan more patients and shorten the path from initial to final diagnosis. At the same time, MR departments are looking for ways to improve the satisfaction of patients and referring physicians.
AI-based image reconstruction can accelerate MR exams to increase department productivity and reduce the cost per exam while supporting diagnostic confidence with high-resolution images. The technology also increases MR accessibility for stressed, anxious, or in pain patients. Because it shortens exams and provides first-time-right scans regardless of patient condition, patients spend less time in the scanner, improving their experience.
3. Taking the complexity out of ultrasound measurements
In cardiac care, ultrasound has become an indispensable tool for visualizing and evaluating a patient’s heart function. It is now often the first imaging modality used to diagnose patients. However, the challenge with ultrasound is that it strongly relies on the person operating the system. Certain measurements needed for every echo exam can be time-consuming and have high inter- and intra-user variability.
We can eliminate some of that manual and repetitive labor with AI, just like in some of the other AI in healthcare examples in this overview. AI-based automatic measurements can deliver fast and reproducible echo quantification, improving patient and staff experience. Healthcare professionals can accept or modify measurements based on their clinical assessments, giving them a powerful tool to enhance their expertise while remaining in control of diagnostic decision-making.
4. Helping radiologists read images faster and more accurately
Similarly, AI can support image segmentation and quantification in radiology, enabling radiologists to focus on higher-level interpretation of images. AI can also act as a second set of eyes, supplementing the radiologist’s decision-making by pointing out areas of interest or incidental findings they may have overlooked.
For example, AI algorithms that scour MR images of the brain for subtle neurological changes over time have been shown to improve diagnostic accuracy in multiple sclerosis patients by 44% while reducing reading times. Similarly, AI-based lung nodule detection can perform nodule search 26% faster, detecting 29% of previously missed nodules compared to manual inspection. For time-pressured radiologists who must interpret more images than ever, such AI-enabled support can make a meaningful difference while benefiting the patient.
5. Supporting multidisciplinary collaboration in cancer care
Beyond analyzing single data sources, there are also examples of AI in healthcare that show it can help connect previously disconnected and disparate patient data – providing novel insights that support healthcare professionals' decision-making.
In cancer care, for example, AI can help integrate information across different clinical domains such as radiology, pathology, EHR systems, and genomics – providing a clear, intuitive view of the patient’s disease state. This can assist multidisciplinary tumor boards in making timely, informed treatment decisions to give every patient the best chance of a positive treatment outcome.
In the future, the intelligent integration of data could give further insight into a patient’s prognosis, supporting the selection of the best care pathway for that particular patient based on an analysis of treatment outcomes for similar patients.
In conclusion, the fusion of healthcare and artificial intelligence represents a paradigm shift in medicine, offering transformative possibilities for improving patient care, operational efficiency, and medical research. By harnessing the power of AI-driven technologies, healthcare stakeholders can unlock unprecedented opportunities to enhance diagnostic accuracy, personalize treatment regimens, and accelerate medical innovation. However, realizing the full potential of AI in healthcare requires navigating ethical, regulatory, and implementation challenges to ensure equitable access, patient privacy, and algorithmic fairness. As we embark on this journey of technological innovation, collaboration among policymakers, healthcare professionals, technologists, and ethicists is essential to harnessing the benefits of AI while upholding ethical principles and safeguarding patient welfare in healthcare delivery.
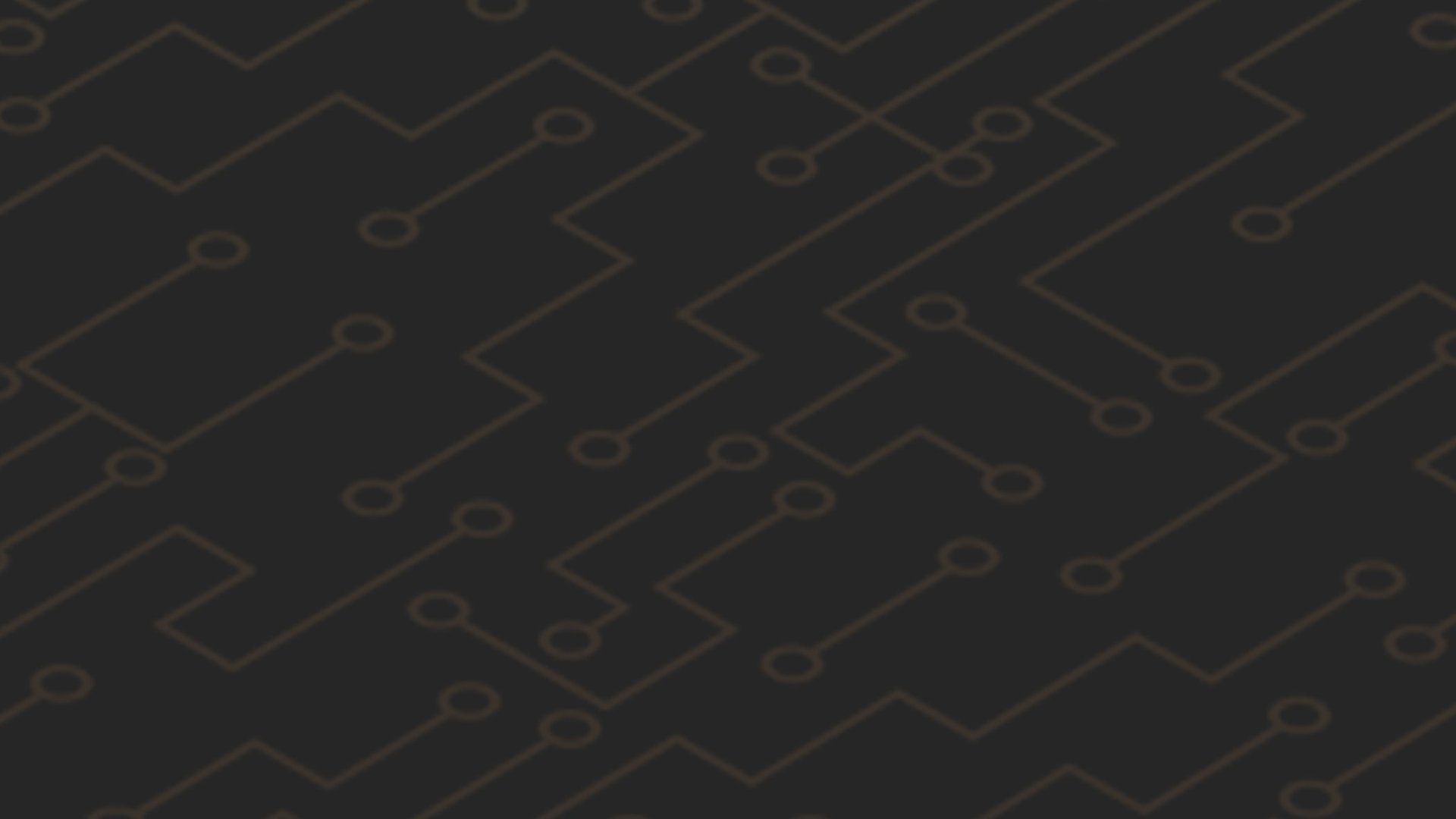
Subscribe and follow to stay up-to-date!
Contact us for any concerns or question through: ainexusais@gmail.com