A New Era Of Healthcare: Artificial Intelligence In Diagnostic Radiology
Discussing the rising implementation of artificial intelligence in the field of radiology and how it is used.
HIGHLIGHTSARTICLES
Abdulrahman Atef
7/2/20244 min read
With the potential to automate repetitive tasks, analyze complex data, and make life-saving decisions, Artificial Intelligence offers a bright future full of possibilities for the field of medicine, specifically Radiology, a branch of medicine that uses imaging technology to diagnose and treat disease.
Many use Convolutional Neural Networks (CNNs) to perform medical image analysis. Trained using large datasets of medical images, CNNs can identify patterns and anomalies in images with a high degree of accuracy. As a result, CNNs have achieved expert-level performance in radiology.
AI-powered medical imaging can help radiologists in several ways, such as:
Image Classification: AI algorithms can classify medical images into different categories, such as normal or abnormal, based on predefined criteria. This can help radiologists prioritize cases and reduce the time they take to make a diagnosis
Image Segmentation: AI algorithms can segment medical images into different regions of interest, such as tumors or organs, which makes it easier for radiologists to analyze and interpret the images
Image Detection: AI algorithms can detect abnormalities in medical images that human radiologists may miss, leading to earlier and more accurate diagnoses
Artificial vs Human Intelligence
The rise of artificial intelligence (AI) in medicine, and particularly in radiology, is becoming increasingly prominent. Its impact will transform the way the specialty is practiced and the current and future education model.
While most earlier AI methods have led to applications with subhuman performance, recent deep learning algorithms can match and even surpass humans in task-specific applications (see the figure below).
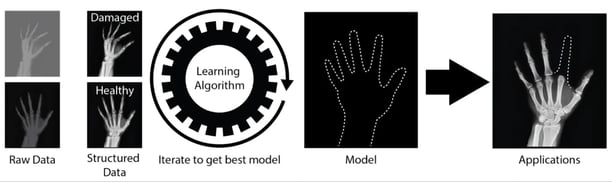
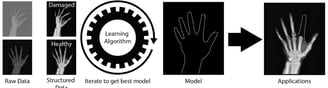
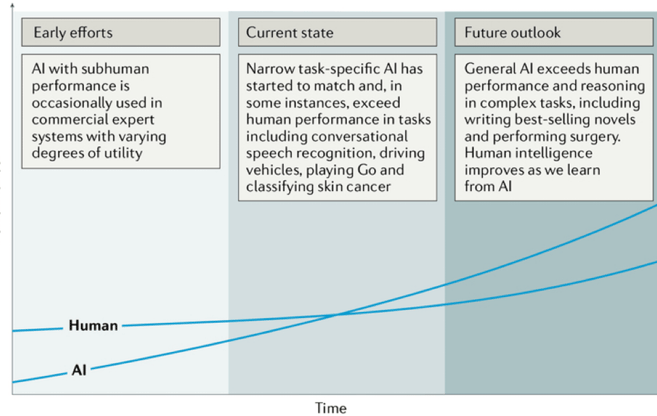
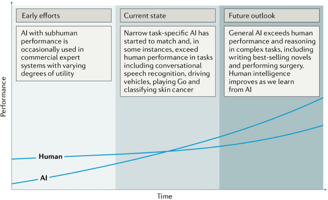
This plot outlines the performance levels of artificial intelligence and human intelligence starting from the early computer age and extrapolating into the future. Early AI came with a subhuman performance and varying degrees of success. Currently, we are witnessing narrow task-specific AI applications like the ones used in radiology that can match and occasionally surpass human intelligence and efficiency.
Examples of clinical application areas of artificial intelligence in radiology
Thoracic imaging:
Lung cancer is one of the most common and deadly tumors. Lung cancer screening can help identify pulmonary nodules, with early detection being lifesaving in many patients. Artificial intelligence (AI) can help in automatically identifying these nodules and categorizing them as benign or malignant.
Abdominal and pelvic imaging:
With the rapid growth in medical imaging, especially computed tomography (CT) and magnetic resonance imaging (MRI), more incidental findings, including liver lesions, are identified. AI may aid in characterizing these lesions as benign or malignant and prioritizing follow-up evaluation for patients with these lesions.
Colonoscopy:
Colonic polyps that are undetected or misclassified pose a potential risk of colorectal cancer. Although most polyps are initially benign, they can become malignant over time. Hence, early detection and consistent monitoring with robust AI-based tools are critical.
Mammography:
Screening mammography is technically challenging to interpret expertly. AI can assist in the interpretation by identifying and characterizing microcalcifications (small deposits of calcium in the breast).
Brain imaging:
Brain tumors are characterized by abnormal tissue growth and can be benign, malignant, primary, or metastatic; AI could be used to make diagnostic predictions.
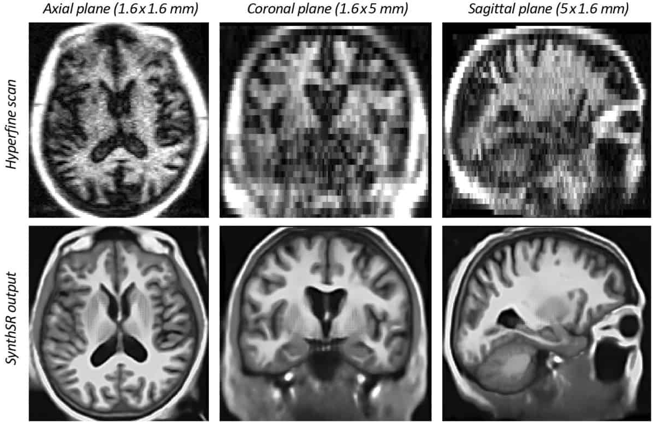
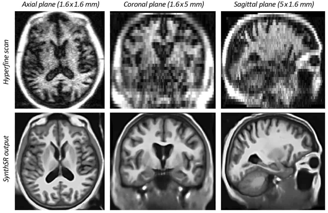
Artificial Intelligence Methods In Medical Imaging
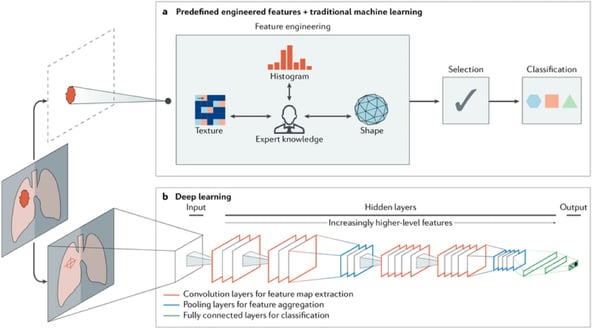
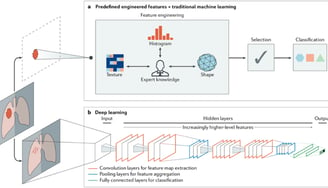
This schematic outlines two artificial intelligence methods for a representative classification task, such as diagnosing a suspicious object as benign or malignant. The first method relies on engineered features extracted from regions of interest based on expert knowledge. Examples of these features in cancer characterization include tumor volume, shape, texture, intensity, and location. The most robust features are selected and fed into machine learning classifiers. The second method uses deep learning and does not require region annotation — rather, localization is usually sufficient. It comprises several layers where feature extraction, selection, and ultimate classification are performed simultaneously during training. As layers learn increasingly higher-level features, earlier layers might learn abstract shapes such as lines and shadows, while other deeper layers might learn entire organs or objects. Both methods fall under radiomics, the data-centric, radiology-based research field.
The increasing application of artificial intelligence in medicine is revolutionizing medical practices and the healthcare industry. With the potential to automate repetitive tasks, analyze complex data, and make life-saving decisions, AI offers a bright future full of possibilities for the field of medicine. Its use is already being seen in radiology, pathology, and drug discovery, with the potential for further growth and application. Overall, the use of AI is set to transform the way medicine is practiced.
Thanks for reaching the end of this article. If you have any questions, please reach out to us directly on our LinkedIn account or through our email: ainexusais@gmail.com
Some of the sources we used to write this article:
Visser J. What the Radiologist Should Know about Artificial Intelligence
Ahmad Z., Rahim S., Zubair M., Abdul-Ghafar J. Artificial Intelligence (AI) in Medicine, Current Applications and Future Role with Special Emphasis on Its Potential and Promise in Pathology: Present and Future Impact, Obstacles Including Costs and Acceptance among Pathologists
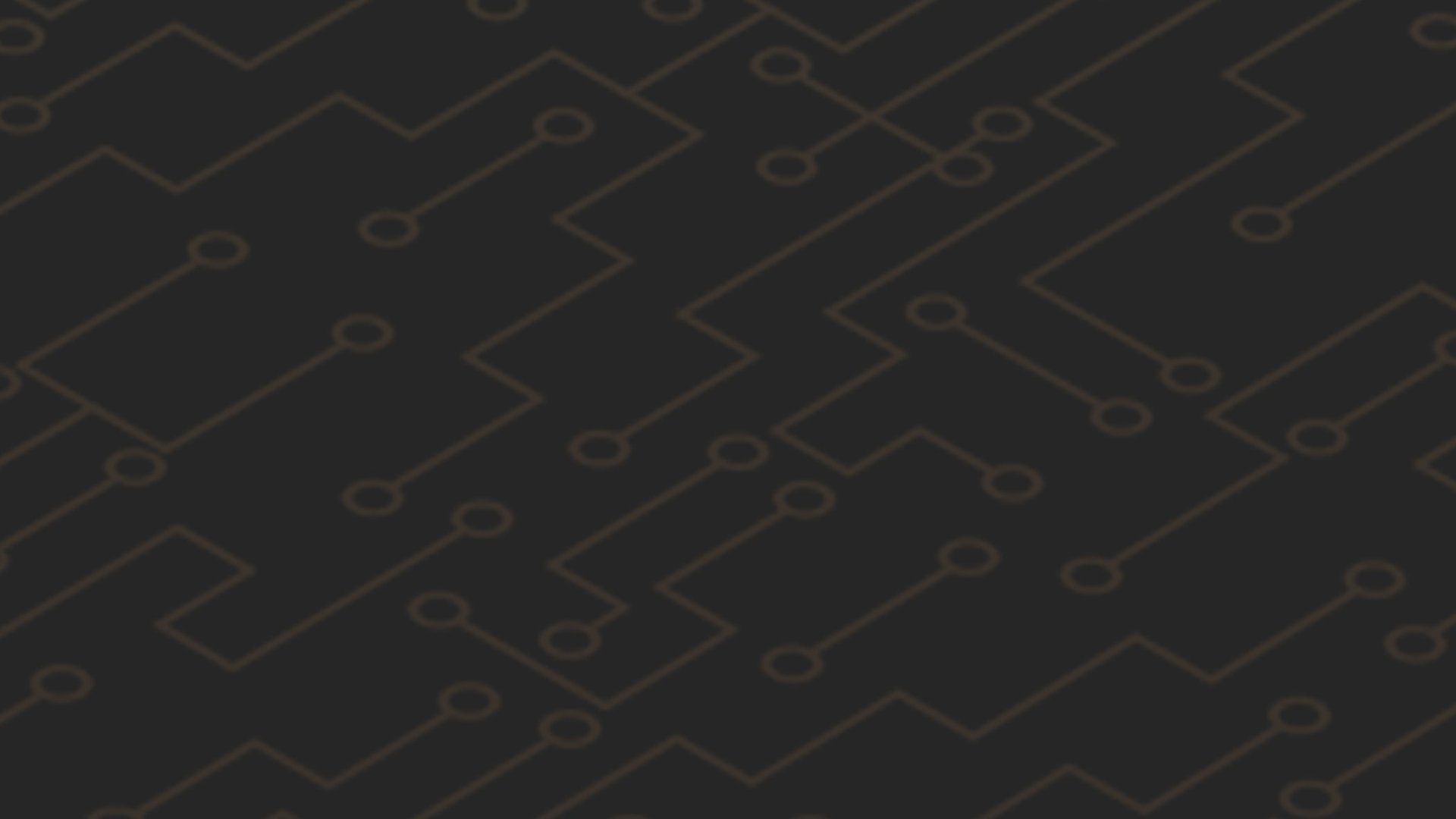
Subscribe and follow to stay up-to-date!
Contact us for any concerns or question through: ainexusais@gmail.com